Tensor Cloud for AI/ML with NVIDIA GPU
Cloud with Kubernetes and NVIDIA GPU H100 / L40s / L4 with tensor cores for runnig artificial intelligence and machine learning (AI/ML) workloads.
The platform combines the world's most powerful tensor accelerators NVIDIA H100 / L40s / L4 and an integrated technological stack based on VMware vSphere, VMware Tanzu Kubernetes Grid and NVIDIA AI Enterprise. Tensor accelerators provide unattainable performance for other technologies on the tasks of machine learning and productive inference.
Get a powerful and reliable GPU Cloud for AI/ML service that provides 100% cost predictability, economic efficiency and a significant reduction in time from idea to implementation.
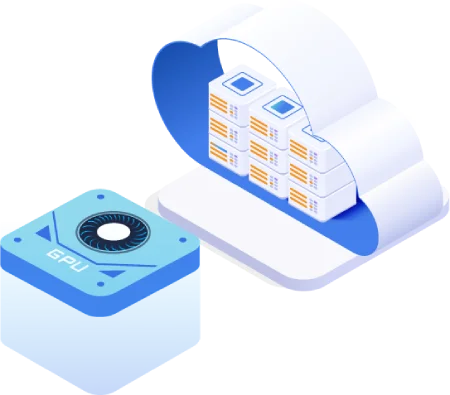
Tensor Cloud Prices
NVIDIA GPU-accelerated instances are optimized for AI/ML and HPC workloads. Using GPU virtualization technology from VMware and NVIDIA, you can order the H100/L40s/L4 in parts (1/8, 1/4, 1/2, or the whole) to meet your workload needs.
The hourly price is approximate based on 730 hours per month and the exchange rate of 1$ = 41 UAH. Prices are exclusive of VAT. Payment is made in UAH per month at constant prices without reference to foreign exchange rates.
Card | GPU RAM, GB | vCPU | vRAM, GB | Price GPU + instance, $/hr | Price GPU + instance, грн/міс |
---|---|---|---|---|---|
¼ NVIDIA L4 | 6 | 2 | 12 | 0,16 | 4 832 |
½ NVIDIA L4 | 12 | 4 | 24 | 0,32 | 9 664 |
NVIDIA L4 | 24 | 8 | 48 | 0,65 | 19 328 |
⅛ NVIDIA L40s | 6 | 2 | 12 | 0,26 | 7 728 |
¼ NVIDIA L40s | 12 | 4 | 24 | 0,52 | 15 456 |
½ NVIDIA L40s | 24 | 8 | 48 | 1,03 | 30 912 |
NVIDIA L40s | 48 | 16 | 96 | 2,07 | 61 824 |
⅛ NVIDIA H100 | 10 | 2 | 24 | 0,65 | 19 328 |
¼ NVIDIA H100 | 20 | 4 | 48 | 1,29 | 38 656 |
½ NVIDIA H100 | 40 | 8 | 96 | 2,58 | 77 312 |
NVIDIA H100 | 80 | 16 | 192 | 5,17 | 154 624 |
14 days Free Trial
How does NVIDIA H100 differ from other AI GPUs?
NVIDIA H100 is a state-of-the-art GPU specially designed for AI and high-performance computing. It is built on the Hopper architecture, which delivers substantial performance improvements over previous generations, including those based on Ampere A100. The key distinction of the H100 lies in its immense computing power, achieved through a greater number of Tensor Cores and the incorporation of new AI acceleration technologies, such as the Transformer Engine.
One of the key advantages of H100 is its capacity to efficiently process deep learning models with a vast number of parameters. With enhanced memory bandwidth and FP8 support, this AI GPU accelerates the training and inference of neural networks, thereby reducing the cost of data processing. In comparison to A100, the new accelerator exhibits 3-4 times greater performance in generative AI tasks, making it the optimal choice for working with models like GPT and other large language models.
Another key difference of the H100 is its enhanced energy efficiency and integration with scalable computing clusters. The new architecture supports fourth-generation NVLink, facilitating swift data transfer between multiple GPUs, which is essential for distributed computing. Furthermore, the H100 provides specialised solutions for cloud providers and data centres, making it an optimal choice for companies utilising scalable AI solutions.
The NVIDIA H100 represents a groundbreaking solution for machine learning, high-performance computing, and cloud services. Its benefits in speed, energy efficiency, and support for emerging computing formats render it an essential tool for companies involved in the development and implementation of advanced AI technologies.
Which tasks are best suited for NVIDIA L4 and L40S?
NVIDIA L4 and NVIDIA L40S are contemporary graphics accelerators tailored for AI, graphics, and video processing. They concentrate on various use cases, delivering high performance and energy efficiency in cloud environments and data centres. With support for hardware acceleration of AI and robust codecs for video analytics, they are becoming essential components of modern computing platforms.
NVIDIA L4 is optimised for broadcasting, video processing, and multimedia content management tasks. It supports hardware decoding and encoding of AV1, H.264, and H.265 video, making it an excellent solution for streaming, video conferencing, and video analytics. With its power efficiency and high memory bandwidth, the L4 is also well-suited for cloud services, providing an optimal balance between performance and cost.
The NVIDIA L40S is designed for resource-intensive tasks, including generative artificial intelligence, rendering, and accelerating neural network computing. This graphics processor provides excellent performance in handling complex 3D graphics scenes, simulations, and machine learning. With increased video memory and support for modern AI algorithms, the L40S serves as a robust tool for companies operating in the domains of computer vision, design, and digital content creation.
Thus, the NVIDIA L4 and L40S address different yet complementary tasks. The L4 excels in video processing and cloud services, while the L40S is suited for complex calculations in artificial intelligence and rendering. Choosing between them depends on the specific requirements of the project and the computational needs.
Why Choose GPU Cloud for AI/ML?
Cloud GPUs represent an optimal solution for artificial intelligence and machine learning tasks, enabling the use of high-performance graphics processors without the necessity of purchasing expensive equipment. Unlike local servers, cloud GPUs offer flexibility and scalability, allowing users to swiftly increase computing power as their needs evolve. This is particularly crucial for training complex neural networks, which require substantial computational resources and prompt access to them.
One of the primary advantages of GPU cloud is its availability and cost-effectiveness. Purchasing and maintaining your own servers with powerful graphics accelerators requires significant investment, whereas cloud solutions allow you to pay for resources as you utilise them. This reduces costs and makes artificial intelligence technologies accessible even to small companies and startups. Moreover, cloud providers offer pre-built software stacks and optimised environments for working with frameworks such as TensorFlow, PyTorch, and JAX.
Another significant reason to opt for GPU cloud is its ease of management and integration with other cloud services. Modern virtual platforms offer tools for automating experiments, monitoring performance, and distributing training of models. This greatly accelerates the development and testing of solutions, allowing teams to concentrate on research and optimising algorithms, rather than focusing on infrastructure.
Therefore, utilising GPU cloud for AI/ML is the optimal choice for those seeking maximum performance at minimal cost. The flexibility, availability, and ease of management render cloud graphics accelerators essential for the development and implementation of advanced artificial intelligence technologies.
How can renting a GPU server help to reduce infrastructure costs?
Renting a GPU server enables you to considerably lower infrastructure costs by offering high-performance computing power without the necessity of purchasing and maintaining costly equipment. In contrast to acquiring your own graphics accelerators, renting a GPU cluster offers flexibility in resource utilisation, allowing companies to scale computing in accordance with their current needs.
One of the primary financial benefits of GPU leasing is the absence of capital investment. Acquiring powerful graphics processors such as NVIDIA A100 or H100 necessitates considerable investment, along with expenses for their installation, cooling, and power consumption. Conversely, leasing enables you to pay solely for the actual use of resources, which is particularly advantageous for companies that do not require constant computing power. This strategy aids in optimising the budget by directing funds towards product development and research rather than sustaining expensive infrastructure.
Another crucial aspect of GPU leasing is the ability to access the latest technology without the necessity of regularly upgrading hardware. In the rapidly evolving realm of artificial intelligence and high-performance computing, hardware quickly becomes outdated. By utilising cloud or dedicated virtual GPU servers, companies can consistently operate on state-of-the-art hardware, thereby evading the need for upgrades and depreciation. Furthermore, leasing frequently includes technical support and the establishment of optimised environments, which alleviates the pressure on the IT department.
Consequently, renting GPU servers serves as an effective solution for companies aiming to reduce infrastructure expenses and access advanced technologies. The flexibility in resource management, absence of capital expenditures, and availability of modern graphics accelerators render this option ideal for businesses involved in artificial intelligence, computer vision, data analytics, and other computationally intensive tasks.
What are Tensor Cores and how do they work?
Tensor Cores are specialised compute cores developed by NVIDIA to accelerate tensor operations, which are extensively used in machine learning and high-performance computing. They first emerged in the Volta architecture and have since become a critical component of NVIDIA GPUs, greatly enhancing deep learning performance. Unlike traditional CUDA cores, which handle floating point and integer operations, Tensor Cores are optimised for matrix calculations, rendering them indispensable for working with neural networks.
The fundamental principle of Tensor Cores is to execute numerous matrix operations concurrently. They support mixed-precision formats (FP16, FP8, INT8), facilitating significant acceleration of data processing without a notable loss of accuracy. This is particularly crucial when training and inferring deep neural networks, where a high volume of matrix multiplications can hinder the process. By employing Tensor Cores, machine learning models can be trained several times more quickly than with traditional methods.
Another key advantage of Tensor Cores is their integration with libraries such as TensorRT and cuDNN, which simplifies their use in real-world applications. They facilitate the optimisation of neural network computations, thereby reducing latency and energy consumption. This makes them sought after not only in scientific research but also in commercial solutions. As a result, Tensor Cores have become the standard for modern graphics accelerators utilised in artificial intelligence, computer vision, and generative models.
Therefore, Tensor Cores serve as a powerful tool for accelerating matrix calculations, enabling effective solutions to machine learning problems. Their high performance, mixed-precision support, and integration with software libraries establish them as a crucial component of modern GPUs tailored for AI computing and scientific research.